Improving Streamflow Projections in Changing Climate Conditions
Uncertainty quantification of machine learning model prediction improves trustworthiness.
April 21, 2023
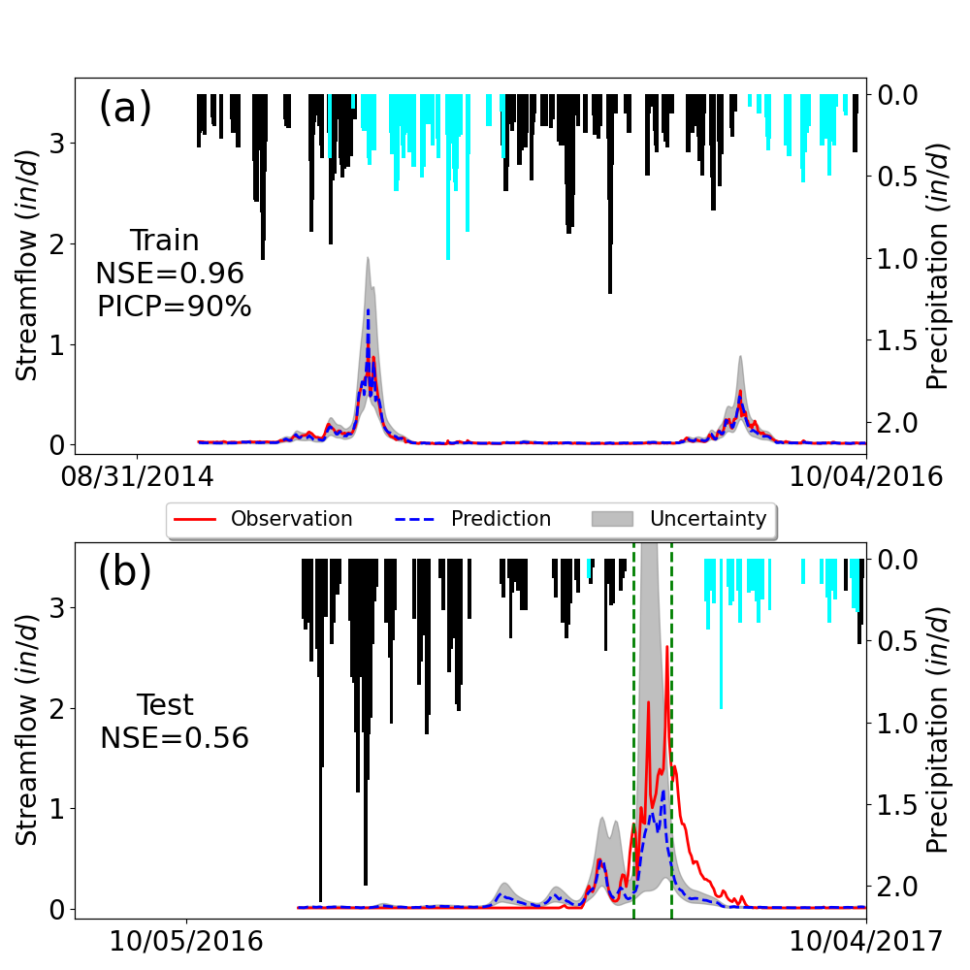
The developed uncertainty quantification method accurately quantifies the machine learning model prediction uncertainty of the streamflow on Rock Creek.
[Reprinted under a Creative Commons Attribution 4.0 International License (CC BY 4.0) from Liu, S., et al. "Uncertainty Quantification of Machine Learning Models to Improve Streamflow Prediction Under Changing Climate and Environmental Conditions." Frontiers in Water 5, 1150126 (2023). DOI:10.3389/frwa.2023.1150126.]
The Science
Machine learning (ML) models, particularly long short-term memory (LSTM) networks, perform well for streamflow prediction in conditions that are similar to training data but become uncertain when extrapolating to new conditions. This study developed a novel uncertainty quantification (UQ) method that trains three neural networks to calculate prediction intervals of an LSTM model. Large uncertainty is signaled when attempting to extrapolate to new conditions, which helps avoid overconfidence in projections in changing climate and land use conditions.
The Impact
Flood prevention and water management require reliable projections of streamflow in changing environmental conditions. These projections require UQ to help guide decision-making. This study developed a novel method to quantify uncertainties in ML-based projections. Those uncertainties are generated by data noise and when extrapolating to novel conditions outside the range of training data. The method is computationally efficient, robust in performance, and can be broadly applied in ML-based simulations to improve the trustworthiness of projections in changing environmental conditions.
Summary
A team of researchers from Oak Ridge National Laboratory developed a novel UQ method to quantify ML model prediction uncertainty. The method calculates prediction intervals by training three neural networks for selected low and high quantiles in addition to the mean. The approach accurately quantifies the predictive uncertainty caused by the data noise and identifies out-of-distribution (OOD) data in a non-stationary condition to avoid overconfident predictions. The UQ method was applied to the snow-dominant East River Watershed in the western United States and to the rain-driven Walker Branch Watershed in the southeastern United States. For the prediction data which have similar features as the training data, the method quantifies the predictive uncertainty with the desired confidence level. For OOD data where the LSTM network may fail to make accurate predictions, the UQ method produces a reasonably large uncertainty estimate to indicate that the results are not trustworthy.
Principal Investigator
Dan Lu
Oak Ridge National Laboratory
lud1@ornl.gov
Program Manager
Jennifer Arrigo
U.S. Department of Energy, Biological and Environmental Research (SC-33)
Environmental System Science
jennifer.arrigo@science.doe.gov
Paul Bayer
U.S. Department of Energy, Biological and Environmental Research (SC-33)
Environmental System Science
paul.bayer@science.doe.gov
Jay Hnilo
U.S. Department of Energy, Biological and Environmental Research (SC-33)
Data Management
Justin.Hnilo@science.doe.gov
Funding
The work was supported by the ExaSheds project and the Watershed Dynamics and Evolution Science Focus Area within the U.S. Department of Energy’s (DOE) Biological and Environmental Research (BER) Program.
References
Liu, S., et al. "Uncertainty Quantification of Machine Learning Models to Improve Streamflow Prediction Under Changing Climate and Environmental Conditions." Frontiers in Water 5 1150126 (2023). https://doi.org/10.3389/frwa.2023.1150126.